Technology governance is the under-appreciated factor in technology competition. The societies that harness new technologies to improve their standards of living, grow their economies, and strengthen their security will be best positioned to win a long-term contest. New technologies can also be deeply destabilizing, harm individuals and communities, undermine confidence in government, and engender a backlash that stifles innovation. The governments that encourage technology innovation while ensuring it is accomplished safely, responsibly, and with public support will be at a competitive advantage and offer a model for the rest of the world. Success in striking the balance between driving innovation and minimizing harm hinges on the norms, rules, frameworks, regulations, and laws that determine how technologies are applied.
Artificial intelligence is the technology posing the most immediate, vexing, and wide ranging set of governance challenges across the world today. In that respect, getting AI governance right is the key to getting tech governance right. The United States must develop a compelling and workable AI governance model or risk living in a world in which technologies that deeply affect our everyday lives do not reflect our values and where we cede innovation leadership to others. By 2025, in the absence of American leadership, much of the world might very well be living either under digital norms dictated by the authoritarian CCP or under overly-restrictive regulatory regimes set up in response to AI skepticism and fear.
In a systems contest to demonstrate the superiority of democracy, using AI to broadly benefit society will be a competitive advantage. The useful applications of AI are wide-ranging and expanding. AI is enhancing decision making across many areas – for cybersecurity, factory and supply chain optimization, medical image processing, and more. AI is enabling physical platforms to become increasingly autonomous, and the trend toward more sophisticated autonomy is clear. Consider warehouse robotics, precision agriculture, self-driving cars, and ocean transport. Most significantly, AI is accelerating scientific discovery and engineering, for example with protein folding, drug discovery, fusion magnet controls, and breakthroughs in astronomy.
To capitalize on the potential of AI for social benefit, the United States must govern AI systems wisely. Shaping the development and use of AI will require calibrating the full range of governance mechanisms, regulatory and non-regulatory, to strike the right balance. We should not let the pendulum swing too far in the direction of a singular focus on minimizing risk. Such an extreme would dampen innovation by reducing investment in new inventions and adding impediments to new adoption. We also must not let the pendulum swing too far in the direction of “move fast and break things” when real harm is a possibility. This extreme increases the risks of harm and could produce a backlash leading to a singular focus on risk minimization with heavy-handed regulation. The right balance requires informed risk tradeoff decisions so that we maximize the benefits while minimizing the harms based on the specific uses of AI.
In authoritarian states, these tradeoff decisions are made by the state with no need to gain the consent of the governed. Liberal democracies require respect for human rights and the rule of law. The United States can find a competitive advantage if it illustrates a model of AI governance that upholds democratic values and norms while also supporting innovation, economic growth, and national security interests. The challenge is how to create a broadly shared understanding of the way forward on technology governance that is rights-protecting and innovation-enhancing.
Given the breadth of its impact, competing approaches to technology governance are playing out most sharply in AI. Governments across the ideological spectrum are grappling with how to influence AI advances to serve their societies1. The PRC is developing an authoritarian approach2. Some of its regulations may look good on “paper,” and indeed mirror regulations that could be adopted by democracies. But Beijing’s methods of social control reveal its true priorities. In contrast, the EU is attempting to create a democratic model that leverages its regulatory strength to create an ecosystem of trust, alongside investments to create an “ecosystem of excellence.”3 But in our assessment, the EU’s regulatory gamble might stifle innovation due to compliance costs and associated burdens for SMEs.4 The UK’s AI governance approach is a deliberate attempt to strike a balance – maximizing growth and competition, driving innovation, and protecting its citizens’ rights; it represents an alternative to the EU model but has not yet been implemented through laws and regulations.5
The United States cannot promote AI advancements that support its vision of democratic ideals without comprehensive national strategies for governing them at home.
It is time for an American approach that is innovation-friendly and still responsive to legitimate concerns about harms of AI applications. Today, however, the United States does not yet have a coherent strategy to present to the world. The United States cannot promote AI advancements that support its vision of democratic ideals without comprehensive national strategies for governing them at home. These strategies need to garner public confidence in technology and governing institutions, promote innovation, and lay the foundation for maximizing the opportunities presented by AI-enabled systems.
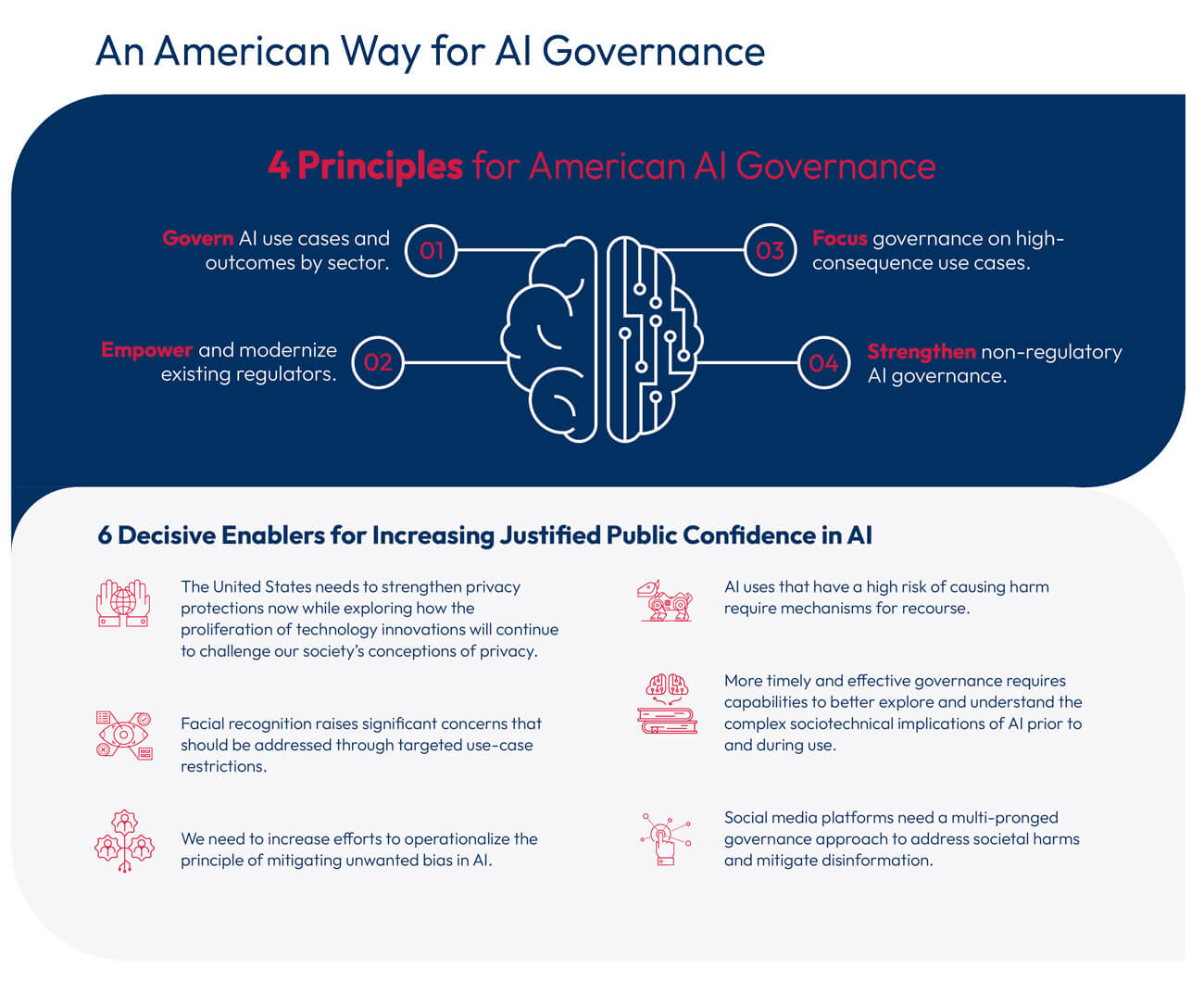
Four Principles for American AI Governance
An American way of AI governance should be guided by four principles:
First, govern AI use cases and outcomes by sector. The risks and opportunities presented by AI are inextricably tied to the context in which it is used. Currently, the United States is pursuing sector-specific efforts to regulate AI by adapting existing regulatory frameworks and agencies to address new issues introduced by the adoption of AI.6
Although some advocate for broader cross-sector AI regulation7, trying to assign regulatory oversight across broad use cases to a centralized regulator would introduce a range of problems and inefficiencies.8 A sector-specific approach is consistent with past American regulatory successes. However, information about AI applications and lessons learned should still be shared across sectors.9 Existing structures and processes to facilitate this cross-sector communication should be encouraged and expanded.10
Second, empower and modernize existing regulators. The United States should rely on its existing constellation of sector-specific regulators,11 which can be equipped to address new regulatory needs raised by AI. Existing regulatory bodies have the sector expertise that allows for tailoring rules, ensuring AI governance complements existing non-AI governance, and assessing impacts.12 However, we must identify the resources these agencies currently lack to address regulatory challenges posed by AI. Because existing regulatory bodies were created in a different technology era, the United States needs to modernize them for the new AI era.13
This might require adding AI-specific talent, infrastructure, or training. This will happen only if political leadership prioritizes AI at existing regulatory agencies at the federal, state, and local levels.14 In addition, the United States needs to develop and use tools and mechanisms to better understand the technical and economic feasibility, including a cost/benefit analysis, of potential regulation.
Because existing regulatory bodies were created in a different technology era, the United States needs to modernize them for the new AI era.
Non-regulatory mechanisms can address non-critical AI challenges and harms, and in certain circumstances can be more effective than regulation in shaping AI development and use.
Third, focus governance on high-consequence use cases. Because it is impractical to govern every AI use or outcome, the United States should shape those AI technologies that will be most impactful. The United States needs a framework for categorizing AI use cases as having the potential to cause major harm, such as widespread discrimination or due process violations. Identifying these types of high-risk AI use cases and enforcing restrictions will require legislative and/or executive actions. There are multiple existing risk characterization frameworks being developed both domestically and internationally that could inform the U.S. national approach.15
Fourth, strengthen non-regulatory AI governance. In addition to its regulatory guardrails, the United States should strengthen and nurture its robust non-regulatory ecosystem.16 Civil society participation in governance is an American strength, and non-regulatory mechanisms draw on this by exerting power through incentives and public opinion. Non-regulatory mechanisms can address non-critical AI challenges and harms, and in certain circumstances can be more effective than regulation in shaping AI development and use.17 They allow for the flexibility necessary to adjust to a technology that is rapidly evolving and allow for participatory experimentation that can be calibrated and adapted to the maturity of AI. This intentional focus on iterative learning and refinement reflects the reality that any specific mix of AI governance mechanisms is a snapshot in time; technology advances and our understanding of the interactions between AI systems and society must be reflected in our AI governance adaptation. AI and the social environment in which AI is used will continue to change. Governance is ongoing and not an endpoint.
Six Decisive Enablers for Increasing Justified Public Confidence in AI
Public mistrust could dampen adoption of socially beneficial AI-based systems.18 This mistrust may also encourage an aggressive regulatory stance based on a precautionary, risk-averse approach rather than a more nuanced and informed risk-tradeoff approach.19 The United States needs a viable public policy reflecting broader consensus in six key areas to help obviate this skepticism in AI and clear the innovation pathway.
- The United States needs to strengthen privacy protections now while exploring how the proliferation of technology innovations will continue to challenge our society’s conceptions of privacy.
The creation of data tied to individuals is inherent to our digital world. Data on individuals can be sorted and analyzed to produce inferences about larger groups.20 As part of a larger national data strategy (described in Chapter 2), we need to protect the right to privacy, ensure that networks and services that rely on data are trustworthy and secure, and enable data use and sharing for economic and social good. The United States should prioritize three actions to improve data privacy protections: (1) Pass federal privacy legislation. The collection, combination, and use of data cuts across sectors and thus requires broad federal legislative protections; (2) Prioritize research in privacy enhancing capabilities.21 A critical concern is the ability of AI-enabled data fusion and inference to pull sensitive insights from disparate, seemingly innocuous datasets; and (3) Promote sustained public dialogue on the future of privacy. Society’s reasonable expectations of privacy are changing as technology advances and our privacy future needs more engagement from all parts of our society to grapple with difficult questions.22
- Facial recognition raises significant concerns that should be addressed through targeted use-case restrictions.
As with other applications of AI, facial recognition is neither inherently good nor bad, and it is used beneficially in a variety of contexts.23 Concerns about facial recognition center on privacy and consent, accuracy and bias, and questionable uses and misuses.24 The United States should govern the use of facial recognition technology, not ban the technology.25 There are many positive uses for facial recognition technologies, and polling shows that public support remains significant.26 However, without targeted restrictions, this technology risks undermining democratic values, for example, by compromising privacy and exacerbating biases.27 Legal authorities should account for different risk levels and use contexts between the commercial, government, and law enforcement communities.28 The American approach to facial recognition regulations should be sector-specific and enforced by existing sector regulators.
We need to protect the right to privacy, ensure that networks and services that rely on data are trust-worthy and secure, and enable data use and sharing for economic and social good.
- We need to increase efforts to operationalize the principle of mitigating unwanted bias in AI.
One of the strongest motivations for public distrust in AI is its power to amplify existing bias in some cases.29 There is considerable national and global focus on addressing the issue of fairness and bias in AI, highlighted in published AI ethics principles from government, industry, academia, and civil society.30 Despite this attention and investment, there is clearly a long way to go to establish justified confidence in the mitigation of unwanted bias. Progress is needed to: (1) Increase multi-disciplinary focus on ways that AI systems are affected by and affect social constructs, assumptions, and individual and collective behavior.31 (2) Implement more intentional use of all levers of governance both before and after AI adoption. (3) Increase research and adoption of ways that AI can expose and help mitigate aspects of bias.32 (4) Better understand the ways in which governance of non-AI parts of a system can reduce biases that manifest in the AI system.33 A specific use of AI may help expose and reduce bias or it may amplify it, but it is often a mirror that reflects the challenges and opportunities in the broader society in which it operates.34
- AI uses that have a high risk of causing harm require mechanisms for recourse.
Governing AI outcomes that have a high risk of causing harm requires ensuring that those affected by these AI systems have recourse to learn why they were negatively impacted and ways to address it.35 This would contribute to justified public confidence in AI use by ensuring that people have the option to challenge potentially capricious or erroneous results. It also implies some design constraints on the development of AI that pose a high risk of causing harm. Those adversely affected by AI should have the opportunity to appeal the outcomes of an AI-based system. In many cases, existing regulatory frameworks can be adapted for this purpose.36
- More timely and effective governance requires capabilities to better explore and understand the complex sociotechnical implications of AI prior to and during use.37
Governing emerging technology requires making informed decisions about tradeoffs between priorities. Government and industry lack widespread access to capabilities to explore the interaction of multiple AI systems and human agents that operate together and how the interaction impacts society. The typical reactive approach is to address complex societal effects of an AI system after deployment. We need technical and non-technical capabilities to better anticipate implications of AI on society and the societal influences on these technologies before and during their deployment.
There are emerging tools and techniques that can be used to develop the capabilities to anticipate societal impacts. Modeling techniques are starting to demonstrate the capabilities necessary to explore the complex dynamics of the interaction of multiple AI systems and human agents over time and their effects on the society in which they are introduced.38 The value of these capabilities is not to “predict the future,” but to enable intentional exploration of potential interactions between an AI system and society to anticipate potential outcomes requiring attention prior to deployment.39
There is urgency to this challenge. AI-enabled decision systems, a critical subset of AI systems, are increasingly used in high-consequence systems. Without better anticipatory capabilities, we will always lag in our ability to mitigate unintended societal harm or we will avoid adopting transformative beneficial capabilities because of reluctance to risk unknown consequences.
We need technical and non-technical capabilities to better anticipate implications of AI on society and the societal influences on these technologies before and during their deployment.
- Social media platforms need a multi-pronged governance approach to address societal harms and mitigate disinformation.
AI-enabled social media platforms have become part of daily lives, and dramatically changed our societies. These platforms provide information at an unprecedented volume and scale, revolutionizing how individuals interact. The ability to instantaneously interact with users around the world alters public engagement in an unprecedented way.40 But these same platforms destabilize societies and enable the spread of disinformation at a global scale.41
Multiple elements contribute to the proliferation of disinformation on social media platforms. The harms caused by social media platforms are the result of both business models and user choices.42 Therefore, a national strategy to mitigate disinformation should include several components: (1) Direct resources and expertise into developing digital literacy programs for the most affected populations. (2) Develop public trust by working with local news media outlets to bolster dissemination of credible information at the community level and sponsor research into methods for overcoming barriers created by the “Liar’s Dividend.”43 (3) Cooperate with allies and partners, by sharing and learning best practices that could inform U.S. disinformation policy. (4) Find ways to lower toxicity and increase transparency, for example by requiring content publishers to watermark or otherwise label their content with information related to source origin; increase algorithm transparency by varying degrees for users, civil society, and oversight authorities; and allow users to control what type and how much of a certain type of content they see. (5) Continue to develop privacy-protecting tools like unique pseudonyms, which can be useful for detecting and stopping automated bots that propagate disinformation at scale.44
1. For a broad comparison see Johanna Weaver and Sarah O’Connor, Tending the Tech-Ecosystem: Who should be the tech-regulator(s)?, Australian National University at 6-7 (2022).
2. China has issued a series of policy documents and policy pronouncements on its governance regime for AI that aligns with its state interests. See Matt Sheehan, China’s New AI Governance Initiatives Shouldn’t Be Ignored, Carnegie Endowment for International Peace (2022); Katharin Tai, et al., Translation: Guiding Opinions on Strengthening Overall Governance of Internet Information Service Algorithms, DigiChina (2021). They tend to focus on regulating the provider organization or outcome with the resulting benefit that they are applicable regardless of technology and resilient to tech changes. See Helen Toner, et al., Translation: Internet Information Service Algorithmic Recommendation Management Provisions (Draft for Comment) Aug. 2021, DigiChina (2021).
3. On Artifcial Intelligence – A European Approach to Excellence and Trust, European Commission at 3 (2020). The European Union has issued the GDPR and its AI Act is geting closer to being set into law, both of which tilt toward protectionism and arguably do not prioritize innovation. Despite their weaknesses, these laws have the advantage of being the frst broad regulatory regime rooted in democratic values that are presented globally. In the past, evidence has shown that the EU’s head start on transnational legislation made it a model for the rest of the world, thereby expanding its regulatory impact. See Jonathan Keane, From California to Brazil, Europe’s privacy law has created a recipe for the world, CNBC (2021). In the present case, it is unclear whether the AI Act’s so-called “Brussels efect” will dictate companies’ new AI standards. However, another factor that could indirectly lead to the same outcome is compliance costs. It might be costlier to have diferent operating standards, rather than making the AI Act the standard – especially in the absence of competing legislation.
4. See Evangelos Razis, Europe’s Gamble on AI Regulation, U.S. Chamber of Commerce (2021) (“According to one study sponsored by the European Commission, businesses would need as much as $400,000 up front just to set up a ‘quality management system.’ Few startups or small and medium-sized businesses can pay this price of admission into the AI marketplace, let alone the additional costs associated with compliance.”) (citing Study Supporting the Impact Assessment of the AI Regulation, European Commission (2021)). While the EU also intends to focus on strengthening innovation, only time will tell whether SME compliance burden concerns are addressed.
5. The UK’s national strategy for AI touches on a broad breadth of challenges: emphasizing the need for talent and R&D provisions and mitigating social harm, while ensuring the uptake of innovation adoption. National AI Strategy, UK Secretary of State for Digital, Culture, Media and Sport (2021).
6. Examples include the Food and Drug Administration’s rulemaking for machine learning (ML) as a medical device and good ML manufacturing processes, the Federal Aviation Administration’s policy on how AI in safety-critical avionics should be addressed in regulation, and the Federal Trade Commission’s application of its current regulatory authorities to new commercial uses of AI. Artifcial Intelligence and Machine Learning in Software as a Medical Device, U.S. Food and Drug Administration (2021). Good Machine Learning Practice for Medical Device Development: Guiding Principles, U.S. Food and Drug Administration (2021). Chris Wilkinson, et al., Verifcation of Adaptive Systems, U.S. Federal Aviation Administration (2016). Elisa Jillson, Aiming for Truth, Fairness, and Equity in Your Company’s Use of AI, U.S. Federal Trade Commission (2021).
7. Anton Korinek, Why We Need a New Agency to Regulate Advanced Artifcial Intelligence, Brookings (2021); S.1896, Algorithmic Justice and Online Platform Transparency Act (2021) (levying requirements for algorithms regardless of sector or use case); H.R.6580, Algorithmic Accountability Act of 2022 (2022) (requiring impact assessments for decision making systems across sectors, such as healthcare, loan approval, and hiring systems).
8. Mariano-Florentino Cuellar & Aziz Z. Huq, The Democratic Regulation of Artifcial Intelligence, Knight First Amendment Institute at Columbia University (2022) (“The idea of a single, centralized regulator with wide-ranging power over a new, general-purpose technology doesn’t seem efective either from a political-economy, a historical, or even a constitutional perspective.”).
9. For example, some of the lessons learned about governing the safety-critical aspects of autonomous vehicles are likely relevant to concerns about governing other safety-critical uses of AI in embedded systems such as medical AI.
10. As an example, the National Artifcial Intelligence Initiative Act of 2020 states that the [National AI Initiative Commitee shall] “coordinate ongoing artifcial intelligence research, development, and demonstration activities among the civilian agencies, the Department of Defense and the Intelligence Community to ensure that each informs the work of the others.” See Pub. L. 116-283, William M. (Mac) Thornberry National Defense Authorization Act for Fiscal Year 2021, 134 Stat. 3388 §5101(a)(4) (2021).
11. Adoption of AI under existing regulatory authorities is consistent with OMB Memo M-21-06. See Memorandum from Russell T. Vought, Director of the Ofice of Management and Budget, Guidance for Regulation of Artifcial Intelligence Applications, Executive Ofice of the President of the United States (2020).
12. Sachin Waikar, Algorithms, Privacy, and the Future of Tech Regulation in California, Stanford Institute for Human-Centered AI (2022) (quoting Jennifer Urban) (“Regulation aims to provide guardrails, allowing a robust market to develop and businesses to fourish while refecting the needs of consumers. Regulators need to understand the business models and whether their actions would be ‘breaking’ something in the industry.”).
13. For example, “[t]hough the FDA can trace its origins back to the creation of the Agricultural Division in the Patent Ofice in 1848, its origins as a federal consumer protection agency began with the passage of the 1906 Pure Food and Drugs Act.” When and Why Was FDA Formed?, U.S. Food & Drug Administration (2018). Software-controlled medical devices and machine learning in clinical diagnostics were obviously not in the initial charter. As traditional software and later machine learning began to play roles in regulated systems, the FDA adapted to address the new regulatory challenges. Artifcial Intelligence and Machine Learning (AI/ML)-Enabled Medical Devices, U.S. Food & Drug Administration (2021). The FAA has a similar history. When established in 1958, digital avionics were not a factor in regulation and oversight. As the technology advanced and began to play a role in aviation, the FAA adapted and extended its regulatory scope to include digital avionics. Digital Avionics Systems – Overview of FAA/NASA/Industry-wide Briefng, NASA (1986); Emma Helfrich, DO-178 Continues to Adapt to Emerging Digital Technologies, Military Embedded Systems (2021).
14. Insurance regulation, for example, is largely at the state level in the U.S., and there is a clear focus on what has to be adapted to refect growing use of AI in the insurance sector. See e.g., Azish Filabi & Sophia Dufy, State Insurance Legislators at the Forefront of Regulating AI, The American College of Financial Services (2022).
15. Three pre-existing risk characterization frameworks—the EU AI Act (proposed), European Commission (2021), AI Risk Management Framework (initial draft), National Institute of Standards and Technology (2022), and Framework for Classifcation of AI Systems, OECD (2022)—may be useful in providing such guidance on risk-assessment. Each framework takes a slightly diferent approach, has diferent goals, and thus yields diferent implications for how to assess risk.
16. These include voluntary standards and best practices, self-governance, independent auditing, journalism, advocacy, philanthropy, policy research, legal recourse, government contracting requirements, government funding, incentives, waivers, exemptions, Congressional public hearings and investigations to inform potential legislation, and government-issued policy guidance or frameworks.
17. Non-regulatory mechanisms have traditionally been insuficient to address high risks of harm (e.g., digital avionics and medical devices have consistently been regulated instead of being governed solely by companies).
18. Lee Rainie, et al., How Americans Think about Artifcial Intelligence, Pew Research Center (2022).
19. Adam Thierer, The Proper Governance Default for AI, Medium (2022) (“The logic animating the precautionary principle refects a well-intentioned desire to play it safe in the face of uncertainty. The problem lies in the way this instinct gets translated into law and regulation. Making the precautionary principle the public policy default for any given technology or sector has a strong bearing on how much innovation we can expect to fow from it. When trial-and-error experimentation is preemptively forbidden or discouraged by law, it can limit many of the positive outcomes that typically accompany eforts by people to be creative and entrepreneurial. This can, in turn, give rise to diferent risks for society in terms of forgone innovation, growth, and corresponding opportunities to improve human welfare in meaningful ways.”).
20. Martin Tisné, The Data Delusion: Protecting Individual Data Isn’t Enough When the Harm is Collective, Stanford Cyber Policy Center (2020).
21. The U.S. Government is already taking important steps to accelerate responses to evolving privacy threats. The Fast Track Action Commitee (FTAC) on Advancing Privacy Preserving Data Sharing and Analytics, led by the White House’s Ofice of Science and Technology Policy (OSTP) and the Networking and Information Technology Research and Development (NITRD) Program, is a strong efort to drive advances in the privacy technology space. Advancing Privacy-Preserving Data Sharing and Analytics, NITRD Program (last accessed 2022). The United States and United Kingdom announced they would collaborate on innovation prize challenges for privacy-enhancing technologies. Press Release, US and UK to Partner on Prize Challenges to Advance Privacy-Enhancing Technologies, The White House (2021). The United States and European Union Trade and Technology Council (TTC) also highlighted PETs as a priority area for cooperation. FACT SHEET: U.S.-EU Trade and Technology Council Establishes Economic and Technology Policies & Initiatives, The White House (2022).
22. Karen Hao, Coronavirus is Forcing a Trade-of Between Privacy and Public Health, MIT Technology Review (2020); Derek Korte, 3 Privacy Tradeofs That Might Be Worth It, WIRED (2015).
23. Some examples include unlocking personal mobile devices, accessing ATMs, passing through airport or event security, and checking into hotels. See e.g., Where is Facial Recognition Used?, THALES (last accessed 2022).
24. GAO-20-522, Facial Recognition Technology: Privacy and Accuracy Issues Related to Commercial Uses, U.S. Government Accountability Ofice (2020); Katam Raju Gangarapu, Ethics of Facial Recognition: Key Issues and Solutions, Learn Hub (2022).
25. As an example of a ban, Microsoft is restricting the use of its facial recognition tool and will stop ofering automated tools to predict a person’s gender, age, and emotional state. James Vincent, Microsoft to Retire Controversial Facial Recognition Tool that Claims to Identify Emotion, The Verge (2022).
26. Lee Rainie, et al., Public More Likely To See Facial Recognition Use By Police as Good, Rather Than Bad for Society, Pew Research Center (2022); Tom Simonite, Face Recognition Is Being Banned – but It’s Still Everywhere, WIRED (2021). Even when there is consensus on a particular use (e.g., combating child exploitation), nuanced challenges remain around how the data was gathered for that application (e.g., via internet scraping of social media). See Richard Van Noorden, The Ethical Questions That Haunt Facial-Recognition Research, Nature (2020).
27. Sam duPont, On Facial Recognition, the U.S. Isn’t China – Yet, Lawfare (2020).
28. A 2021 Center for Strategic and International Studies report proposes a useful set of principles to shape federal rules: Permissible Use, Transparency, Consent and Authorization, Data Retention, Autonomous Use, Redress and Remedy, Oversight and Auditing, Algorithmic Review, and Training Data. See James Lewis, Facial Recognition Technology: Responsible Use Principles and the Legislative Landscape, Center for Strategic and International Studies (2021).
29. Distrust of Artifcial Intelligence: Sources & Responses from Computer Science & Law, Daedalus (last accessed 2022).
30. AI ethics principles that reference bias include AI Principles: Recommendations on the Ethical Use of Artifcial Intelligence by the Department of Defense, Defense Innovation Board (2019); Principles of Professional Ethics for the Intelligence Community, Ofice of the Director of National Intelligence (2014); Memorandum for the Heads of Executive Departments and Agencies: Guidance for Regulation of Artifcial Intelligence Applications, Ofice of Management and Budget (2020); Jessica Fjeld, et al., Principled Artifcial Intelligence: Mapping Consensus in Ethical and Rights-Based Approaches to Principles for AI, Berkman Klein Center (2020); Anan Mahmood, Tackling Bias in Machine Learning Models, IBM (2022); Responsible AI Practices, Google AI (last accessed 2022); Dana Pessach & Erez Shmueli, A Review on Fairness in Machine Learning, ACM Computing Surveys (2022). Eforts to operationalize the principle of mitigating unwanted bias include recommendations from the NSCAI. Key Considerations for Responsible Development and Fielding of Artifcial Intelligence, National Security Commission on Artifcial Intelligence (2021). The proposed National AI Initiative Act of 2020 requires R&D to mitigate bias. See H.R.6216, National AI Initiative Act of 2020 (2020). Multiple states have made mitigating unwanted bias a priority. See Legislation Related to Artifcial Intelligence, National Conference of State Legislatures (2022).
31. Andrew Selbst, et al., Fairness and Abstraction in Sociotechnical Systems, Conference on Fairness, Accountability, & Transparency (FAT) ‘19 at 60 (2019) (“[A] sociotechnical frame recognizes explicitly that a machine learning model is part of a sociotechnical system, and that the other components of the system need to be modeled. By moving decisions made by humans and human institutions within the abstraction boundary, fairness of the system can … be analyzed as an end-to-end property of the sociotechnical frame.”). In an algorithm used to manage the health of populations, it was determined that the disparity was not a problem with bias in the training data or a faw in the model; it was due to complex societal factors that afect the healthcare interactions of black and white patients in the U.S. that could not be anticipated or understood by looking at the data and model in isolation. See Ziad Obermeyer, et al., Dissecting Racial Bias in an Algorithm Used to Manage the Health of Populations, Science (2019).
32. AI also ofers a chance to signifcantly reduce bias and unfairness by making it explicit and correctable over time. Jennifer T. Chayes, How Machine Learning Advances Will Improve the Fairness of Algorithms, Hufington Post (2017).
33. For example, the mitigation of biases in predictive policing systems is going to require governance of policing practices, not just the AI systems that inform those practices.
34. Rachel Metz, AI Made These Stunning Images. Here’s Why Experts Are Worried, CNN Business (2022).
35. Responsibility, Recourse, and Redress: A Focus on the Three R’s of AI Ethics, IEEE Technology and Society Magazine at 86 (2022) (“In the context of AI, recourse can be determined as the mechanisms by which a stakeholder (either infuencing or impacted) informs responsible persons or organizations of an unexpected, unfair, or unsafe outcome. …. In the example of a denial of a service or payment, there must be clear guidance identifying the responsible stakeholders, how to contact them, and the right, under relevant regulation, to challenge and ask for reconsideration of the decision process and rectifcation if an error has been commited.”).
36. As an example of adapting existing regulatory frameworks, current regulations for consumer protections in credit decisions apply regardless of the use of AI in the decision making. [The Equal Credit Opportunity Act requires] “creditors to provide statements of specifc reasons to applicants against whom adverse action is taken. …. The adverse action notice requirements of ECOA …. , however, apply equally to all credit decisions, regardless of the technology used to make them.” Consumer Financial Protection Circular 2022-03: Adverse Action Notifcation Requirements in Connection with Credit Decisions Based on Complex Algorithms, Consumer Financial Protection Bureau (2022).
37. “Sociotechnical implications: refers to the implications of AI being embedded in larger “sociotechnical systems,” systems that “consist of a combination of technical and social components.” For example, “fairness and justice are properties of social and legal systems like employment and criminal justice, not properties of the technical tools within.” Andrew Selbst, et al., Fairness and Abstraction in Sociotechnical Systems, Conference on Fairness, Accountability, & Transparency at 59-60 (2019).
38. This is analogous to digital twin techniques, which are currently used to simulate physical objects prior to building them in order to explore design alternatives and implications of a selected design (of jet engines, for example). See Maggie Mae Armstrong, Cheat sheet: What is Digital Twin?, IBM (2020).
39. A recent example of this approach is an agent-based simulation to explore the difusion and persistence of false rumors in social media networks. See Kai Fischbach, et al., Agent-Based Modeling in Social Sciences, Journal of Business Economics (2021).
40. See generally, José Van Dijck, The Culture of Connectivity: A Critical History of Social Media, Oxford University Press at 3-23 (2013).
41. David M. J. Lazer, et al., The Science of Fake News, Science (2018); Sander van der Linden, Misinformation: Susceptibility, Spread, and Interventions to Immunize the Public, Nature Medicine (2022).
42. Sara Brown, The Case for New Social Media Business Models, MIT Sloan (2022); Gordon Pennycook & David G. Rand, The Psychology of Fake News, Trends in Cognitive Sciences (2021).
43. The Liar’s Dividend occurs when malignant actors are able to claim valid information as being invalid. It creates an opportunity for malignant actors to potentially further their narratives and objectives by claiming online media is false – which individuals may believe – regardless of the validity of the claim. Robert Chesney & Danielle K. Citron, Deep Fakes: A Looming Challenge for Privacy, Democracy, and National Security, California Law Review at 1785-86 (2019).
44. The creation of unique pseudonyms online helps identify individual users, while also posing dificulties with connecting an individual online user with the real life person. Pseudonymization is known foremost as a data protection technique, especially for storing data per GDPR standards while protecting personal information. See Thomas Zerdick, Pseudonymous Data: Processing Personal Data While Mitigating Risks, European Data Protection Supervisor (December 2021); see also Lee Rainie, et al., The Future of Free Speech, Trolls, Anonymity and Fake News Online, Pew Research Center (2017).