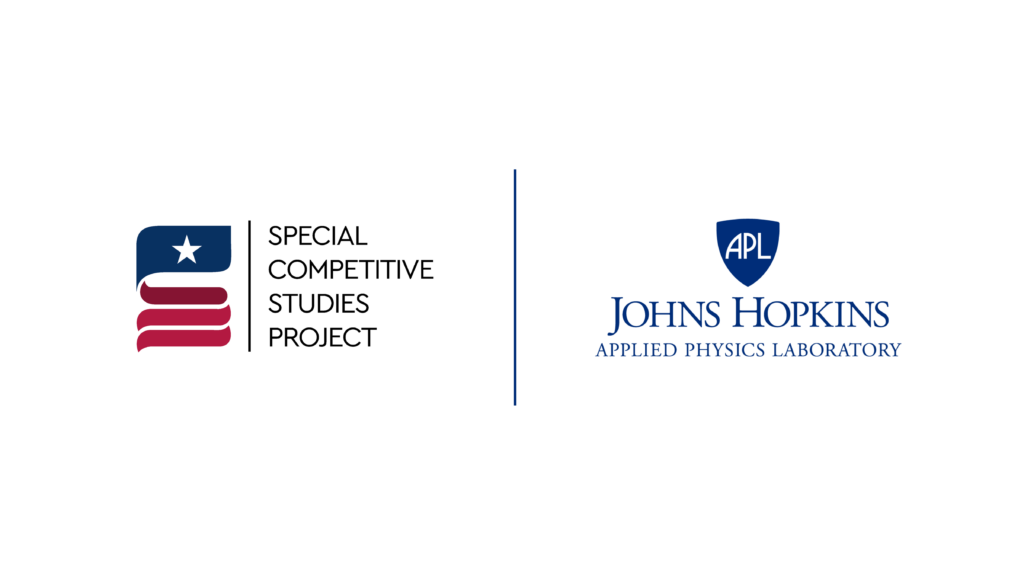
Introduction
The Special Competitive Studies Project (SCSP) in collaboration with the John Hopkins University Applied Physics Laboratory (JHUAPL) developed the “Framework for Identifying Highly Consequential AI Use Cases.”
The genesis of this collaborative effort stems from the comprehensive analysis of the United States’ approach to AI governance in SCSP’s 2022 Mid-Decade Challenges to National Competitiveness report which outlined four pivotal AI governance principles.[1] In Mid-Decade Challenges, SCSP recommends that the United States should leverage its robust sector regulatory expertise to uptake AI regulation and govern AI use cases and outcomes by sector. Regulators also need the necessary resources to adopt AI regulation. A critical AI governance principle is that U.S. regulators cannot regulate every AI use case. Rather, the U.S. regulatory approach must focus their efforts on highly consequential AI use cases, whether beneficial or harmful. This framework operationalizes these principles.
With those principles as the guiding post, this framework is a classification tool that regulators can use to determine on which AI uses and outcomes to focus their regulatory efforts. Without context and awareness of the potential harms and benefits of an AI use case, it is difficult to comprehensively classify the impacts of an AI system. This framework is intended to aid regulators in making an informed initial classification of an AI use case. It does not speak to what regulatory action should be taken, only that an AI use or class of AI use cases will significantly impact society and requires their attention.
The framework is a starting point. It is a template that regulators can modify for their sector-specific needs. However, it will need reiteration, with more input particularly from regulators, to make it more effective and implementable. It should be viewed as a living document.
The framework fits within SCSP’s broader mission to strengthen America’s long-term competitiveness as AI and other emerging technologies shape our national security, economy, and society. To review the breadth of SCSP’s work, visit scsp.ai.
The partnership that produced this report brought together experts and practitioners from across the national security, regulatory, and emerging technology communities through a series of roundtables. SCSP and JHUAPL would like to thank the many government experts and regulators, academics, civil society leaders, and industry experts for their time and insight. It was prepared by SCSP and JHUAPL staff and, as such, may diverge from the opinions of some expert contributors.
Background
Artificial intelligence (AI) is a transformative technology with the potential to revolutionize many aspects of our lives. While AI offers benefits such as improving health, education, productivity, and solving some of the world’s most pressing problems, it also has the potential to be harmful. AI use can result in the spreading of disinformation and discrimination, for example. In order to shape AI for the public good, we must both incentivize the beneficial use of AI while recognizing and mitigating the worst of the societal harms.
AI technologies must be developed and used ethically and responsibly. This requires governing AI, using both regulatory and non-regulatory mechanisms, in ways that align with established democratic values. At the same time, an American approach to AI must balance innovation with regulation.
To achieve these objectives, the Special Competitive Studies Project provided four AI governance principles in its first report, Mid-Decade Challenges to National Competitiveness.[2] These principles are:
- Govern AI use cases and outcomes by sector. The risks and opportunities presented by AI are inextricably tied to the context in which it is used. Therefore, sector-specific governance is the best approach to balancing interests to achieve optimal outcomes.
- Empower and modernize existing regulators. Existing regulatory bodies were created in a different technology era. The United States needs to empower and modernize its key regulators, and energize their engagement for the new AI era.
- Focus governance on high consequence use cases. It is impractical to govern every AI use or outcome. Regulators should focus their efforts on AI uses and outcomes that will be highly consequential to society, including potential unintended uses, whether beneficial or harmful.
- Strengthen non-regulatory AI governance. Strong, robust non-regulatory governance mechanisms should be used in addition to regulatory guardrails to properly shape AI development and use to ensure flexibility, adaptiveness, and relevance.
With respect to governing through regulation, sector regulators are faced with the challenge of regulating the rapidly evolving field of AI technology. AI systems are becoming increasingly sophisticated and widespread, and it is difficult to regulate every system and use case. Some AI development and use cases pose a potential for significant negative or positive impacts on society, and thus, warrant more attention than others. As a result, regulators need to be strategic about which AI use cases and outcomes they focus their regulatory efforts upon.
Multiple frameworks exist for assessing and mitigating risks associated with AI. While these mechanisms are effective for their particular missions, there is no widely accepted approach for identifying whether an AI development or use case is of high consequence to society before further regulatory actions are taken.
The United States and our allies and partners need an approach to AI regulation that promotes innovation by regulating AI systems that have the most significant consequences on society. Such an approach would allow for the continued development of beneficial AI uses, while addressing potentially significant harms from development and use.
A risk-based approach to AI regulation that identifies highly consequential AI use cases aligns with democratic values. This approach respects individual rights, liberties, and freedoms and harnesses impactful benefits of AI systems for public good, while protecting individuals and society from the worst of the harms. The draft EU Artificial Intelligence Act (the “EU AI Act”) also takes a risk-based approach to regulation, meaning that it regulates AI systems based on the potential risk of harm they pose to individuals or society.[3] This framework employs a risk-based approach. However, instead of relying on a static list of technologies and applications, this framework takes into account the dynamic nature of AI technologies and provides regulators with flexibility in its application.
An approach to identifying highly consequential AI systems should be both risk-based and flexible. Such an approach should provide justified confidence in AI systems (by supporting trustworthiness & responsibility) for the public, certainty to industry, and flexibility to regulators to apply sector-specific expertise and experience, as different sectors and uses have different risk thresholds. An American approach should also provide insight into potential beneficial impacts of AI development or use on society that regulators can choose to regulate (e.g., incentivize through funding or consider whether a benefit warrants equitable access by the whole of society).
This document sets forth a framework for identifying highly consequential AI use cases (ID HCAI Framework) that might have significant benefits or harms on society. The framework is a tool that can help regulators ensure that the development and use of AI systems align with democratic values. By using this template, regulators can focus their efforts on AI systems that are highly consequential to the public, standardize their approach across sectors, and adapt their approach to the specific needs of different sectors. Additionally, by documenting their processes and decision making, regulators can help to ensure accountability and transparency. This framework template should be adopted and tailored by regulators for sector-specific needs.
Some AI use cases will require regulatory focus, while others will not. This framework aims to help regulators identify AI use cases in the “gray area.” An initial high-level assessment must be made as to whether the AI use case under consideration warrants the resources to conduct a more thorough assessment of whether an AI system is highly consequential. The initial judgment will help determine whether the AI development or use case under consideration has foreseeable harms that could pose a significant impact on health, safety, or fundamental rights, or substantial benefits that should overwhelmingly incentivize the AI development and use. If not, then no further assessment is required and the AI use case is determined to not be of high consequence. Otherwise, the complete framework should be applied to help determine whether the AI use case is highly consequential to individuals or society. A suggested best practice is to document the process and rationale used at every decision point in the ID HCAI Framework.[4] It is also recommended that regulators establish a registry of evaluated AI use cases and their classifications with exceptions (e.g., for national security or justified industry secrecy). The registry should contain a mechanism by which the public can provide input on these evaluated AI system use cases. This will inform the public of assessments and classifications, allowing them to inform regulators of any contextual changes triggered by the continued use of the AI system that may affect periodic reassessments. It will also have the added benefit of informing industry about the regulators’ evaluation process.
The framework interprets AI as computational systems that do some of the predictions, recommendations, classifications, and other decision making that traditionally are in the province of humans.[5] This definition includes systems which are not possible without AI, and those that make use of AI-based components, AI-enabled functions, or AI-derived algorithms. The framework is intended for assessments of AI systems as a whole, vice components, and their concrete impacts on society that result from how they change the context or condition of society. It further proposes that assessments be performed by regulators with input from multi-disciplinary experts,[6] including the public, which is best positioned to evaluate impacts on society. In addition, societal impacts are those resulting both from the use of the AI system as well as its development (e.g., impacts on data workers and from environmental impacts).
Three AI lifecycle points at which the framework can be applied:
- Regulators foresee a new application for AI,[7]
- A new application for AI is under development or proposed to a regulatory body, and
- An existing AI system has created a highly consequential impact that triggers an ex-post facto regulatory review.
The high-level steps to the framework are:
- Preliminary analysis: Determine whether the AI application has foreseeable harms or benefits that could impact, for example, health, safety, or fundamental rights, and consequently may need to be regulated. This is intended to be an initial filter to determine whether a fuller assessment is needed.
- Parallel analysis of harms and benefits: If there is foreseeable harm or benefit, conduct a more comprehensive harms/benefits analysis, which involves performing parallel harm and benefit assessments.
- Enumerate and evaluate the magnitude of foreseeable and actual harms from the AI system development and use.
- Enumerate and evaluate the magnitude of foreseeable and actual benefits from the AI system development and use.
- Final decision on high consequence: Using the magnitude assessment results, determine if the AI use case is of high consequence.
- If yes, a sector-specific regulator must determine how best to take next steps to regulate the AI development and/or use (e.g., whether to create incentives, mitigate harms, or establish bans).
- Periodic reassessment: Periodically monitor sectoral AI use to determine if the list of AI systems identified as highly consequential remains appropriate for that sector given contextual changes and whether revisions to classifications are necessary.
The potential specific harms and benefits are grouped into ten corresponding categories (see Table 1 and Appendix 1). The framework provides specific harms and benefits for each category, as examples, with the recognition that specific harms/benefits will be unique to sectors. Harms and benefits are further characterized by magnitude (e.g., the scope of a harm or benefit). The framework provides factors to calculate the magnitude of an identified harm or benefit. Specifically, harms are characterized by four severity factors and four likelihood factors. Benefits are characterized by four impact factors and two likelihood factors. Lastly, the document offers ways to make high consequence determinations based on the quantification of a system analysis.
Categories of Harms and Benefits
Table 1. A list of ten harm categories (left) and benefit categories (right). Corresponding categories of harm and benefit are identified on the same row.
Table 1. ID HCAI Framework Corresponding Categories of Harms and Benefits
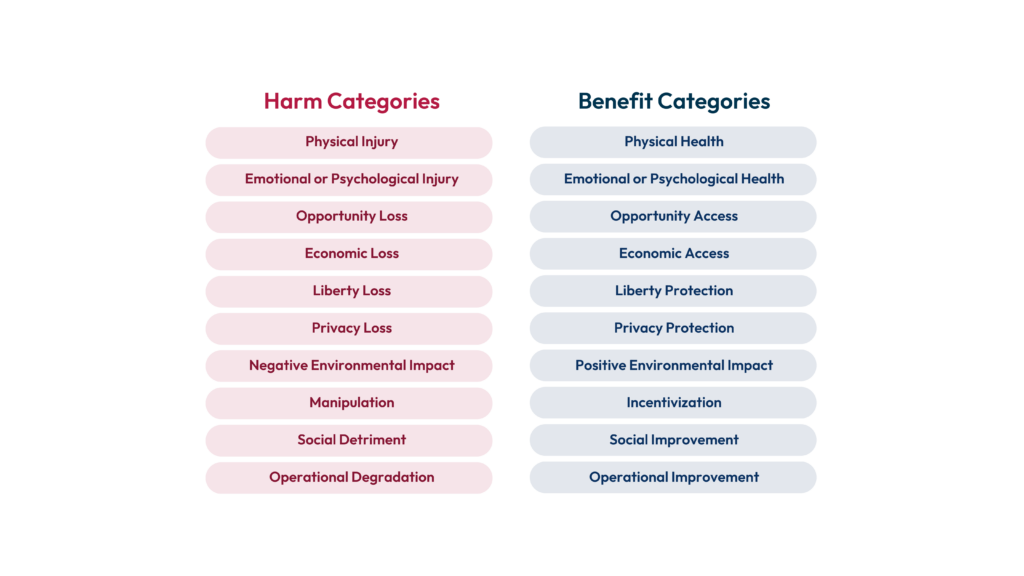
Appendix 1 provides tables of specific harms and benefits for each category, with corresponding descriptions. These tables are intended to guide the framework user (e.g., a sector-specific regulator) through consideration of examples of the types of specific harms or benefits associated with each category. Note that potential violation of fundamental rights is incorporated into the specific harms lists. The tables are meant to be illustrative and not exhaustive lists.
As noted in the high-level steps above, this process begins with an AI development or use case for review. While no application will be completely free of any potential harm and all presumably have some potential benefit, the framework assumes that this process has been employed because the possibility of some significant AI related harm or benefit has been identified as a reasonable outcome. To determine whether the AI development or use should be regulated, a framework user should explore the extent of those potential harms and benefits.
Defining Factors for Characterizing Harm Categories
The framework employs ten categories of harms.[1] Each category includes a non-exhaustive list of specific harms, located in Appendix 1.
The framework user steps through each category of harms to identify and describe relevant specific harms that could result from the development and deployment of the AI application being considered.
For each specific harm, the framework user would evaluate the harm’s potential severity and likelihood, and document any baselines used for comparison that led to the score determinations.[2] The severity of harm factors are scale, scope, disproportionality, and duration. The likelihood of harm factors include probability, frequency, lack of detectability, and lack of optionality. Descriptions for each of these factors are presented in Table 2 and Table 3, respectively.
Table 2. Harm Factors of Severity
Factor | Description |
Scale | How acutely the harm could impact a population or group throughout the AI lifecycle |
Scope | How broadly the harmful impact could be experienced across populations or groups |
Disproportionality | Whether an individual, group, or population is disproportionately affected by the harm over that of other individuals, groups, or populations |
Duration[3] | How long the harmful impact would be experienced by a population or group |
Table 3. Harm Factors of Likelihood
Factor | Description |
Probability | The likelihood the harm could impact a population or group and whether this particular harm has occurred before (e.g., through a similar use case) |
Frequency | How often a population or group would experience the harm |
Lack of Detectability | Likelihood of not discovering and correcting a hazard or failure mode while it remains possible to prevent or mitigate the harm |
Lack of Optionality | Limited individual choice as to whether to be subject to the effects of the technology (e.g., ability to opt-out), such as from minimal human oversight to consider and remedy problems that may be encountered from the AI system |
To analyze magnitudes of harms, based on severity and likelihood factors, a framework user has “scoring” options to assess magnitude at the specific harm level or at the harm category level.
One approach is to employ different rank order categories (e.g., very low to very high, with as many categories in between as desired) with stated descriptions and explanations of the categorization of impacts.[4] The deliberative process should highlight the dimensions and factors with which each harm and harm category was evaluated.
In the alternative, a framework user can employ the Likert scale.[5] For example, the Likert scale could range from 1-5 (with 1 representing “low,” 3 representing “medium,” and 5 representing “high”). Note that the numbers are meant to signal categories that are relative to each other, just as using descriptive categories (e.g., very low to very high). The numbers are not meant to indicate preciseness such that a magnitude “4” is exactly twice as much as a magnitude “2.”
The former approach assumes that a numerical representation is neither possible nor useful, while the latter approach assigns numerical values with some “room for flexibility.” Either approach for scoring the factors allows necessary flexibility because the scales and magnitudes of the different factors will vary for different harms, and the relative judgment of what is a “very high” or “5” score will differ by sector/harm. The factors are named in such a way that a “very high” or “5” score represents a negative aspect of the harm (e.g., “long duration”). The framework user has flexibility in determining the specific weightings for each harm category given the importance of specific categories may vary depending on the sector and context. We instead provide guidance on potential dependencies for each category (see Appendix 1). Some harms may be essentially instantaneous (e.g., a physical harm), while others may extend over time (e.g., psychological harm). Thus, some magnitude factors (e.g., scope) may raise different considerations for those harm categories.
Defining Factors for Characterizing Benefit Categories
As noted in the high-level steps above, a benefits assessment should occur in parallel with the harms assessment. To determine aspects of the AI system that could encourage development and use, the framework user should explore the extent of potential benefits stemming from the use case.
In a similar approach to the harms assessment, the framework employs ten categories of benefits.[6] Each category includes a non-exhaustive list of specific benefits, located in
Appendix 1.
The framework user steps through each category of benefits to identify and describe relevant specific benefits that could result from the development and deployment of the AI application being considered.
For each specific benefit, the framework user would evaluate the benefit’s potential impact and likelihood. The impact factors are scale, scope, duration, and proportionality. The benefit likelihood factors include probability and frequency. Descriptions for each of these factors are presented in Table 4 and Table 5, respectively.
Table 4. Benefit Factors of Impact
Factor | Description |
Scale | How acutely the benefit could impact a population or group throughout the AI lifecycle |
Scope | How broadly the beneficial impact could be experienced across populations or groups |
Duration | How long the beneficial impact would be experienced by a population or group |
Proportionality | Whether a population or group is proportionally affected by the beneficial impact as compared to other populations or groups |
Table 5. Benefit Factors of Likelihood
Factor | Description |
Probability | The likelihood the benefit could impact a population or group and whether this particular benefit has occurred before |
Frequency | How often a population or group would experience the beneficial impact |
To analyze magnitudes of benefits based on impact and likelihood factors at either the specific benefit level or at the benefit category level, a framework user has the same options for implementation as assessing harms – either employing different rank order categories or the Likert scale.
The factors are named in such a way that a “very high” or “5” score represents a positive aspect of the benefit, (e.g., “long duration”). Some benefits may be essentially instantaneous (e.g., physical health), others may extend over time (e.g., psychological health). Thus, some factors (e.g., scope) may raise different considerations for those benefit categories. The framework user has flexibility in determining the specific weightings for each benefits category given the importance of specific categories may vary depending on the sector and context.
Analyzing “Magnitude” for Harms and Benefits
Regulators should have flexibility, based on sector-specific expertise and experience, in analyzing the magnitude of harms/benefits based on the severity, impact, and likelihood factors (“magnitude” analyses). This document suggests that the magnitude analyses of harms/benefits focus at the categorical level.[7] One way to make this analysis is by calculating the magnitude for each specific harm/benefit and calculating an average (weighted) across categories. One advantage of assessing the magnitude factors for each specific harm/benefit in Appendix 1, which are then analyzed at the categorical level, is that this approach provides a comprehensive view of all relevant harms and benefits with a nuanced understanding of the interplay of all the relevant harms and benefits.
Appendix 2 provides an exemplary method of calculating the magnitude analyses for specific harms and benefits, respectively.
Ways to Make High Consequence Determinations
There are many ways a framework user may choose to apply the resulting magnitude values based on their sector-specific expertise and experience. Magnitudes can be considered within a specific category or across categories. Ultimately, any approach will need to determine whether an AI development or use case being assessed is of sufficiently high consequence to warrant continued regulatory focus.
Examples of ways to combine or compare magnitudes include:
- Does any specific harm category have a magnitude greater than threshold X?[8]
- Do one or more harm categories have a magnitude greater than threshold Y?
- Does the total of magnitudes across all harm categories exceed threshold Z?
- Are the total magnitudes across all harm categories greater than the total magnitudes of benefits across all benefit categories?
- Are there one or more harm categories that might overwhelmingly outweigh the assessed categories of benefits?
- Are there one or more benefit categories that might overwhelmingly incentivize development or use, despite the magnitude, number, or type of harm categories identified?
- Does the aggregation of low magnitude level harms/benefits change context to whether the system is considered highly consequential?
Even at the categorical level, regulators should have flexibility in analyzing the magnitude for harms/benefits. For example, a framework user may focus on the most salient harms/benefits as opposed to every possible harm/benefit that exists. The framework also recognizes that even a single harm/benefit or magnitude can signal a high consequence AI use case that requires regulatory focus.
Potential next steps would then depend on responses to the above questions. For example, if the assessed character of the benefits is determined to outweigh the assessed character of the harms, the framework user could specify that development or use of the AI system should proceed, or perhaps even be incentivized. Alternatively, if the assessed character of the harms is determined to outweigh the assessed character of the benefits, then the framework user could specify whether development or use of the AI application should be reconsidered, suggest potential alternatives that have not been considered, or provide recommendations for risk mitigation[9] based on the identified harms. These determinations require sector-specific nuance, taking into consideration aspects that do not have clear answers, such as the willingness to accept the accumulation of minor consequences over time.
Periodic Reassessment of Sectoral AI Use
At the sectoral level, AI impacts should be monitored on a periodic basis to assess if the harmful/beneficial impact(s) of a previously assessed AI system has changed (e.g., environmental change or change in use) or if there has been a contextual change due to socio-techno relationship warranting revisions to the AI classification or regulation as appropriate for that sector. These reassessments may occur at the level of the individual AI system (e.g., an AI-enabled medical device approved by the FDA) or of a class of AI systems (e.g., AI use for credit approval or election campaign use).
Questions a framework user should pose when monitoring an AI system are:
- How is the AI system adapting post classification and deployment to societal and environmental changes within the sector over time? If the AI system does adapt, how regularly will it adapt to such change(s)?
- Framework users must assess, based on unique sector needs, the best points at which to conduct periodic reassessments.
- Framework users must assess, based on unique sector needs, the best points at which to conduct periodic reassessments.
- What aspects of assessment, classification, or regulation need to be revised given this change?
- Whose obligation it is to conduct periodic reassessments. For example, should the obligation rest on the entity responsible for the AI system to report to the regulator (i.e., modeled after reporting recall patterns and defective systems)? Or should the regulator’s responsibility include the periodic reassessments?
Conclusion
This framework aims to provide a tool for regulators to identify which AI use cases and outcomes are or will be highly consequential to society, whether beneficial or harmful. Because it is impractical to govern every AI use or outcome, regulators should focus their efforts on shaping those AI technology uses and outcomes that will be maximally impactful on society. The framework provides latitude for users to identify specific rulesets to employ for determining whether a use case is high consequence, allowing for flexible implementation across sectors. Intra-agency and inter-agency consistency will result from repeatedly applying the same framework template, being transparent through documentation, and building a library of assessments that are publicly available.
Assessments, especially pre-deployment or in early stages of release, might have to be conducted on limited information and based on hypothetical assumptions. New research and data observations post-deployment enable determinations of whether initial classifications are still appropriate and whether revisions to the governance approach are necessary. The framework serves as the first step that a regulator should take in determining if an AI system necessitates further investigation or action. In doing so, the framework also informs policy makers, industry, and civil society on relevant actions to take. It will enable all framework users to focus governance on high consequence use cases, under the assumption that the rapidly expanding application space would overwhelm efforts to address and take action on every use case.
[1] To explore the harms in detail, the framework adapts the Microsoft Types of Harm List to frame AI-related harms that could emerge. See Foundations of Assessing Harm, Microsoft (2022); Types of Harm, Microsoft (2023).
[2] Baselines can include any statutory boundaries for a given sector (e.g., EEOC compliance), or corresponding harms for a non-AI counterpart. Documenting baselines may help regulators develop a library of use cases for comparison.
[3] Example considerations for this factor include the relative difficulty of an individual or group to appeal the outcome from the use of the technology (finality of outcome), difficulty in mitigating a certain risk imparted by the technology (lack of mitigation; refer to NIST RMF), or the minimal ability or speed at which the technology or an affected individual or group can recover or return to normal after a consequential event (lack of resilience). In addition, this factor should assess whether a harm occurs at a low-level continuously over time (e.g., distortion of reality due to prolonged interaction with the AI system) or whether a harm is instantaneous and acute (e.g., malfunction of threat identification system, which triggers a threat neutralization procedure resulting in bodily injury).
[4] Guide for Conducting Risk Assessments, U.S. National Institute of Standards and Technology at Table H-3 (2012).
[5] Rensis Likert, A Technique for the Measurement of Attitudes, Archives of Psychology 140 at 1–55 (1932).
[6] The non-exhaustive list of benefits was compiled from a literature review.
[7] This document suggests analyzing magnitudes at the category level because: (1) specific harms/benefits will be different across sectors; (2) the number of specific harms/benefits and associated factors can result in a comprehensive, but cumbersome number of overall quantifications; (3) analyses at the categorical level account for the interaction across harms/benefits that will alter the impact of any individual harm/benefit assessed independently; and (4) analyses at the categorical level allow for standardization of categories across sectors for comparative analysis and public justified confidence.
[8] Efforts are underway to establish standards, metrics, and norms for AI development and use. See Biden to Push For Government Standards on AI, Politico (2023); U.S. LEADERSHIP IN AI: A Plan for Federal Engagement in Developing Technical Standards and Related Tools, U.S. National Institute of Standards and Technology (2019).
[9] For example, the NIST AI Risk Management Framework provides a voluntary process for managing AI risks prospectively and continuously throughout the AI lifecycle. Artificial Intelligence Risk Management Framework, U.S. National Institute of Standards and Technology (2023).
[1] Mid-Decade Challenges to National Competitiveness, Special Competitive
Studies Project at 87 (2022).
[2] Mid-Decade Challenges to National Competitiveness, Special Competitive Studies Project at 87 (2022).
[3] Tambiama Madiega, Artificial Intelligence Act, European Parliamentary Research Service (2023).
[4] Documentation should include details such as the “moment” in time that an assessment is performed, which will help inform future reassessments and address potential contextual changes (e.g., the societal relationship with the AI system), rationale for making the judgment that there are no foreseeable harms or benefits that necessitate use of the framework, and the decisions made throughout the full assessment.
[5] Artificial Intelligence Risk Management Framework, U.S. National Institute of Standards and Technology at 1 (2023) (“The AI RMF refers to an AI system as an engineered or machine-based system that can, for a given set of objectives, generate outputs such as predictions, recommendations, or decisions influencing real or virtual environments. AI systems are designed to operate with varying levels of autonomy (Adapted from: OECD Recommendation on AI:2019; ISO/IEC 22989:2022).”).
[6] This includes domain experts that can provide research insights (e.g., from universities and Non Governmental Organizations) who understand the evolving nature of relevant/emerging impacts to consider.
[7] In this scenario, regulators should assess AI systems as they would be deployed and used.